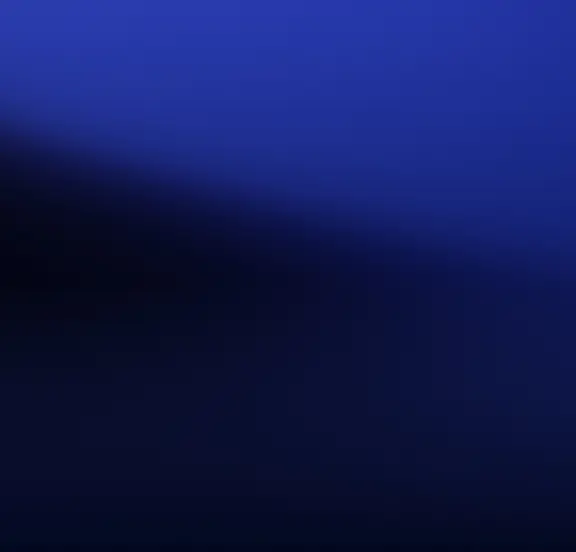
November 17, 2021 7 minutes read
The difference between Artificial Intelligence and Machine Learning
On a general note, innovations and new creations has been prevalent in this age. At the top of it is artificial intelligence. However, machine learning is also closely related to artificial intelligence in some way. That is why it is important to know the relationship between artificial intelligence and machine learning.
As an addition, we will briefly talk about deep learning and the artificial intelligence, deep learning relationship.
The key difference between artificial intelligence and machine learning is that a machine demonstrates the latter. Artificial Intelligence is not a one size fits all concept but requires a nuanced understanding of what constitutes intelligence in a machine, system, or program.
Each has its wide applications in various industries. Therefore, we must clearly understand the difference between them.
Let us consider artificial intelligence.
What is artificial intelligence?
Basically, artificial intelligence (AI) aims to solve complex problems by using smart computer systems to provide responses or perform actions that appear to be intelligent to the human observer.
What are the characteristics of AI?
- It can be categorized as Weak, General, and Strong AI.
- It is concerned with the maximization of successful outcomes using data analysis and pattern recognition.
- It allows a machine to emulate different aspects of human behavior, from speech recognition to language processing, providing responses, and providing different responses based on provided inputs.
- It can work with structured, semi-structured, and unstructured data, depending on the nature of the AI program, the type of data available, and the use-case at hand.
- It can be tailored to perform specific actions, as is the case with chatbots and virtual assistants.
Therefore, these characteristics creates a distinction between artificial intelligence and machine learning.
What is the Classification of AI?
AI can be broadly classified into three distinct categories, namely:
Narrow/Weak AI
Narrow or Weak AI is a field of artificial intelligence that is used for a specific task or purpose. It allows powerful systems to match or exceed human intelligence required for functioning or performing actions that falls within the limited focus of the AI system in question. Examples includes news feed curation features on apps, voice assistants, and AI-powered speech recognition tools.
Strong AI
This, at the moment, is a hypothesized state of AI and is not something the world has currently achieved. The projection is that artificial intelligence will equal human intelligence in terms of cognitive abilities.
Strong AI is also referred to as artificial general intelligence (AGI). Therefore, to reach a point of having strong AI, a system, program, or machine must possess general intelligence and the ability to mimic human intelligence.
Artificial Super Intelligence (ASI)
This is the ultimate stage of artificial intelligence. It is a stage where AI meets and supersedes both general human intelligence and general human cognitive abilities.
Knowing about AI is very important especially now that the world is becoming dominated in AI-powered tech. The knowledge of AI can be a step forward in your career or personal life.
Enough about AI let us talk about machine learning.
Machine Learning
What is machine learning? Are AI and machine learning the same?
Machine Learning (ML) is a subset of AI. It is a learning concept that has fixed outcome goals such as the improvement of a system based on experience. “Improvement” here can be classified in many ways. It could be:
- Correct responses to a customer’s queries
- Correctly identifying different images
- Identifying the presence or absence of cancer cells in patient scans, and more.
The idea is to have machines trained with exposure to relevant datasets for them to have an independent learning technique. Thus, this eliminates the need for machines to be reprogrammed for every new use case or scenario.
For example, an AI program “taught” what cats and dogs look like to determine which of thousands of photos contain cats vs. dogs. However, instead of reprogramming the system from scratch to teach it to differentiate between cats and cars, the system should be able to “learn” that a scanned object is or is not a cat. Then, the system should be able to provide the correct output or response based on experience.
What are the Characteristics of Machine Learning?
The characteristics of machine learning helps to answer the question, “how does machine learning differ from artificial intelligence?
Some of the key characteristics of machine learning are as follows.
- ML allows machines to learn from datasets.
- ML primarily concerns itself with accuracy and patterns.
- ML deals with structured and semi-structured data.
- ML involves the observation and study of experiences and data to identify patterns and make predictions.
What is the Classification of Machine Learning?
Machine Learning can be categorized into the following:
Supervised Machine Learning
This is when an ML algorithm uses predefined categories that are specified by a human operator and tries to group data under those provided categories.
Unsupervised Machine Learning
This is where there are no pre-decided parameters for the system. Instead, the system designs for pattern discovery. That is what constitutes the “learning” of the algorithm.
Semi-Supervised Machine Learning
These models combine both supervised and unsupervised elements in the learning process of the ML system or application.
Reinforcement Machine Learning
This approach rewards the process of trial and error. You can use it when there is a lack of training data so that a system can “learn” each time it receives a correct or incorrect response, rather than learning by processing vast amounts of training data.
Applications of Machine Learning
Effective processing of data and information is possible when ML delivers results by analyzing massive data sets. AI and cognitive technologies can then apply to ML learning models to deliver an effective way to process data and information. From there, the applications of ML only limit the use case and the ingenuity of the user.
Applications of ML technology include:
- Search algorithms and facial recognition systems
- Diagnostic testing (of machines and patients)
- Flagging suspicious digital activity (used by financial systems to prevent fraud)
- Learning user behavior patterns (used in ML-driven targeted ads and data curation), and much more.
AI vs Machine Learning
Are AI and machine learning the same thing?
AI and machine learning are closely connected, however they are not the same thing.
AI covers a wide range of concepts that involves the training of machines to mimic human intelligence in the way they act, think, make decisions and adapt to changes.
Machine learning on the other hand is a subset of AI. AI covers it as one of its concepts. It gives machines the ability to learn independently from the data obtained.
Artificial intelligence vs Machine Learning vs Deep Learning
Artificial intelligence, machine learning and deep learning are three concepts that people often confuse. However related they are, there are some uniqueness between all three that makes them differ. The understanding of AI and machine learning difference is crucial in exploring modern technology.
In earlier sections, we saw highlighted AI vs machine learning. In this section, our focus will be on deep learning.
What is deep learning?
Deep learning is a subset of machine learning and artificial intelligence and gets its data by imitating how humans acquire knowledge. Deep learning models are trained to recognize patterns, texts, pictures, and other forms of data to make predictions and accurate analysis.
Applications of deep learning
Deep learning is a crucial element of artificial intelligence. It is used in AI tools utilized by humans for day-to-day activities. Some of the applications of deep learning includes:
- Facial recognition systems
- Digital AI assistants
- Fraud detection
- Industrial automation
- Text generation
- Chatbots
AI vs Deep Learning
AI and deep learning are very similar. The truth is, they work together.
However, deep learning is a subset of artificial intelligence that depends on multiple layers of artificial neural networks. Deep learning models are trained using these complex neural networks to be able to make accurate predictions, identify objects and carry out other complex actions that otherwise would be possible only with human intelligence.
Therefore, the main difference between AI vs deep learning is that the latter is the driving force for the smooth applications of artificial intelligence in various verticals.
Application of Artificial Intelligence and Machine Learning in Openfabric AI
Openfabric is building a suite of blockchain-based AI tools and applications. These tools can help developers, data users, data providers, and other players find, create, use, and share artificial intelligence and machine learning based tools and applications. As such, these applications are custom-designed for different users, use cases, and industries.
Therefore, by breaking the barriers to access and use, Openfabric is ushering in a new era of AI-based competition and efficiency. The aim is to give users the potential to unlock vast amounts of savings and benefits, both large and small.
Finally, with this, Openfabric AI presents to us a platform that serves as the bridge between AI, machine learning difference.
Learn more by visiting our website.
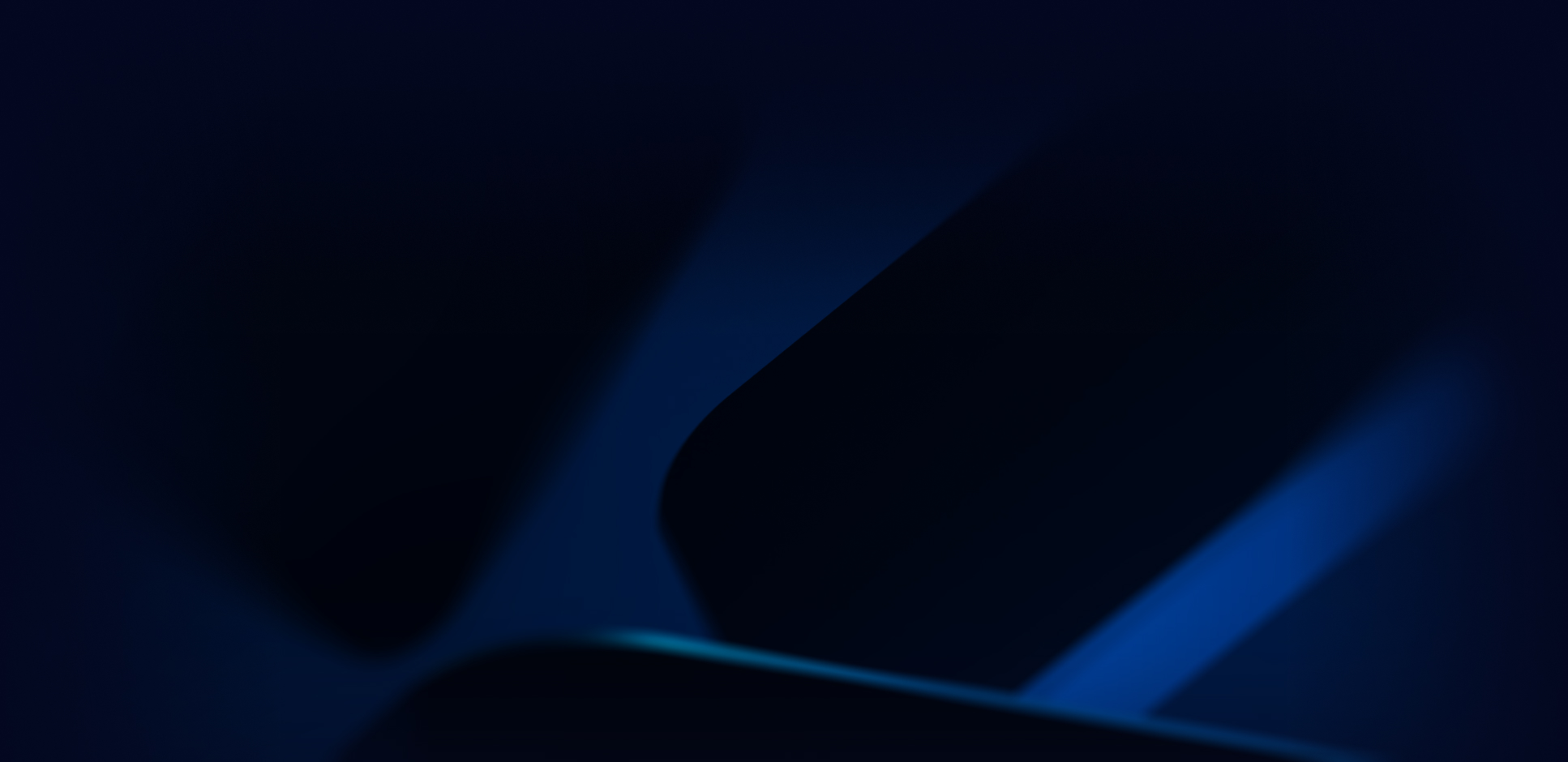