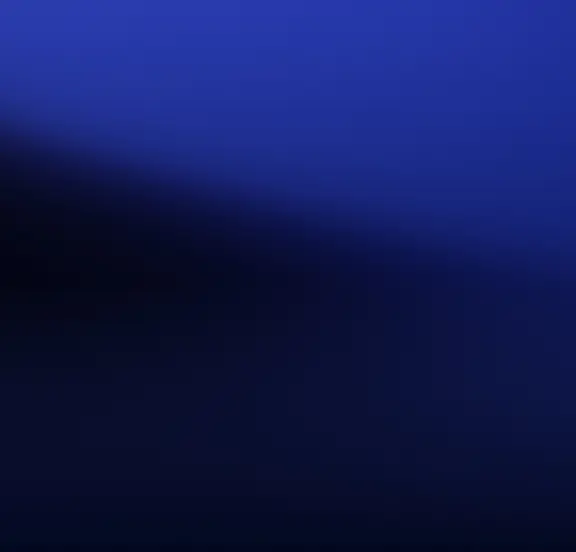
November 11, 2020 12 minutes read
Hello world — The Internet of AI is here!
Today, human intelligence undoubtedly represents the current pinnacle of millions of years’ worth of gradual natural refinement — but while we certainly represent an incredibly crucial moment in this process, what the future holds in store is set to be even more awe-inspiring. However impressive we might be, at the end of the day we still have to acknowledge that we, as individuals, face a daunting task when trying to advance science and knowledge. Furthermore, we — as a global community — are up against serious challenges that our responses are sluggish and unsystematic to.
In recent years, though, the advent of viable AI solutions has promised to give us the tools we need to solve our most significant challenges and begin a technological revolution that will lead to unprecedented human progress occurring at an exponential rate. However, it should also be noted that while AI systems are making steady progress, this promise has not yet materialized — mainly because the intelligent programs are only being used by large firms to solve their domain-specific problems, and because the algorithms are regarded as isolated artifacts.
Openfabric
Openfabric’s mission is to introduce a powerful and exciting paradigm shift in the way people perceive the field of AI, by creating an ecosystem that sees innovation as its most valuable currency. It aims to provide a medium in which multiple types of participants from all kinds of backgrounds can bring their contributions to the table to achieve success and solve their most complex problems by incorporating the power of AI solutions.
Technology-wise, Openfabric is a blockchain-oriented, decentralized environment which offers the following capabilities:
- A decentralized application that connects those who are seeking AI solutions, computational resources and large datasets to those who are willing to provide them.
- A set of CLI and development tools, as well as libraries and frameworks, that facilitate the deployment of algorithms and datasets.
- An SDK that provides access to the underlying platform functions.
- A daemon that allows users to connect to the massively parallel peer-to-peer network which fulfills all the requirements necessary to make the system functional.
On top of its technological infrastructure, Openfabric also innovates in the following directions:
Decentralization — by using the capabilities of blockchain technology and peer-to-peer networks to provide smooth, stable interactions between participants on the platform.
Cooperation towards Innovation — by generating a snowball effect which leads to unprecedented and creative AI solutions to very real, complex issues.
Incentivisation Mechanism — by fostering cooperation between innovators.
Algorithm Rating — by using an adaptive Bayesian model which ensures that users are given access to the best algorithms and datasets the community has to offer.
Protection of Intellectual Property and Regulation Compliance — by running algorithms on data privately, within trusted execution environments.
Enterprise Integration — by providing connectors to the ecosystem, so that enterprises can experience all the benefits that come with leading-edge technology.
All of this will be achieved while also keeping in mind that the actors (stakeholders) should enjoy the User Experience without getting in contact with the underlying complexity of the platform. In the remainder of this text, we will describe the main beneficiaries of the ecosystem, as well as some of the key features that the platform has to offer.
Stakeholders
One of our most valued objectives is to bring the AI revolution to the fingertips of as many users as possible, in the hope that they will go on to form a swarm-like super-consciousness, determined to solve the most intricate problems — and even push the boundaries of knowledge and science in a way that is of real economic impact. In this respect, the ecosystem is designed to facilitate interactions between four types of stakeholders:
- AI innovators use the platform to deploy their own high-quality algorithms by integrating the work of others and then building more complex solutions on top of that work. They will be rewarded whenever their algorithms are executed, either independently or in a mesh of algorithms that collaborate.
- Data providers are the actors who possess vast amounts of data and are willing to be rewarded for sharing it with the community. Their data serves as the fuel which will enable the training of accurate algorithms. Encryption techniques ensure that data will only be accessible inside of trusted execution environments, and so the providers should not be concerned about data leakage.
- Infrastructure providers form the backbone of the decentralized system. They provide the computational resources that will be rented, when programs are being executed. Whenever their resources are selected for running AI algorithms and the job is completed, the infrastructure providers will be rewarded for their contribution.
- Service consumers use the platform to gain new insights by combining data and algorithms, and using the decentralized environment to obtain results. In exchange for this, they are required to pay, in accordance with the features they have used.
Now that you are familiar with the actors who will be the main drivers of progress, let’s move on to the key innovations of the platform.
Decentralization
The Openfabric network will provide a wide range of functionalities, and so it needs to be massively decentralized, in order to ensure that every request is serviced and that there is no single point of failure that compromises the ecosystem. In the current view, the peer-to-peer network provides AI execution facilities, rating mechanisms, and registries for indexing algorithms and data, as well as storage functionality. For this set of functionalities to be readily available, the system must ensure proper coordination between the peers in the network, a feature which can be enabled by a subset of peers that group into a structure similar to that of a layered decentralized operating system (DOS). The DOS provides core logical operations to the rest of the system, analogous to the kernel of a traditional operating system. This decentralized operating system also acts as a decision-maker, using a dynamic consensus mechanism to take on multiple system-wide resolutions in parallel.
Incentivization
An accelerated rate of technological advancement can only be obtained when everyone in the ecosystem is cooperating. And once this point is reached, a mutually beneficial state of equilibrium (Nash equilibrium) emerges.
In order to move the platform into this general direction, Openfabric provides an incentive mechanism which fosters innovator collaboration. It stimulates developers to reuse their peers’ algorithms by combining them in a tree-like structure — in which the more abstract ones are closer to the root, while the simpler ones represent the leaves. The key feature of the mechanism is that every algorithm in the structure will receive a share of the price of the total structure, depending on its position in the tree and how often it is used across the platform. At the same time, though, the end-user will be unaware of this process, because the cost paid is fixed, being determined by the developer.
This strategy is put in place to ensure that the developers are always focused on deploying the next breakthrough algorithm, rather than on deploying different flavors of well-tested and functional ones.
Emergence through AI collaboration. Communication via ontologies.
The incentivization mechanism is only truly efficient if the platform provides AI agent cooperation. Solving a complex problem can only be accomplished by first breaking it down into multiple subproblems. This approach lends itself nicely to algorithm composition, a method through which intelligent algorithms are able to communicate their inputs and outputs (concepts) to other agents that require them. Creating an effective machine learning algorithm is certainly an impressive feat — but weaving together a fabric of such algorithms has the potential to generate the effects of emergence and synergy, through which agents are indeed able to solve manageable subproblems, but the end result is far more valuable than the results of these individual subproblems.
The issue that arises is, how do AI agents achieve communication? Openfabric proposes an efficient model which relies on decentralized ontologies. In this approach, ontologies act as a medium for storing the shared understanding of the concepts that AI agents use and generate. Practically, the ontology behaves as a translator between AI algorithms by offering them a set of concepts through which they can communicate. The ontology model’s efficiency lies within its novel layered architecture:
- The structure layer represents the actual blueprint of all concepts (input or output), as well as the tree-like classification structure that is used to organize them.
- The connection layer contains information about a concept’s location in the decentralized environment, along with its present version and past iterations.
- The encoding layer specifies what standards to use when interpreting the contents of a concept’s instance.
- The defaults layer is used to populate the properties of a concept with their implicit values.
- The validation layer describes the rules that a concept’s properties must obey, so that the concept’s instance can be considered valid.
- The restriction layer describes the interdependencies among properties of a concept and the rules that specify how property values change, in accordance with other property values in the concept.
- The naming layer provides human-friendly, localized names for the ontology concepts.
- The instruction layer provides guidelines, in the case that a human must populate a concept or use the information that it encapsulates.
- The versioning layer is a mechanism that allows the community to modify the current understanding of concepts in the ontologies.
- The template layer provides different, partial views of a concept’s properties.
This architecture splits an ontology into vertical layers and makes communication extremely efficient, because one can operate at various levels of a concept without the need for having to fetch the entire concept from the decentralized network. Additionally, in the structural layer of a concept, one can also specify whether or not a property should be encrypted, therefore making the ontology a suitable candidate for storing sensitive data.
Another key feature of the ontology model is its versioning mechanism, which ensures that the community will play an active part in determining the direction in which the ecosystem evolves. By offering users the ability to modify ontology concepts, they are empowered to make the decisions that are best suited for their own use cases. These decisions will be taken using a combination of a voting mechanism, which will aggregate the preferences of the community members, and a dynamic consensus mechanism, which ensures dispute resolution.
Algorithm Rating
Now that we have determined the manner in which AI algorithms cooperate, let’s present a use-case that sheds some light on another characteristic of the ecosystem. Suppose that a service consumer has a voice recording in language A, and they want a transcript of the recording, but in language B. One way of accomplishing this is by using an algorithm for generating the transcript in language A, and then passing its output to another algorithm which translates the document from language A to language B.
In this scenario, two decisions have to be made, in the form of choosing between different instances of algorithms that perform speech-to-text conversion and automated translation. To help the user make the most informed decision, Openfabric employs Algorithm Rating by harvesting the power of the community. The community uses votes to assign a reputation score to AI algorithms, based on their interaction with those algorithms and the results that they produce. However, users can also act disingenuously in order to game the rating system and obtain unfair advantages (e.g. leave good ratings for their own ineffective algorithms, so as to gain economic benefits). The upside to this is that — as it turns out — disingenuous ratings display statistical patterns that are markedly different from those of honest reviews. Openfabric uses a Bayesian statistical model that is able to detect dishonest algorithm reviews, which further aids the end-users in making informed decisions.
Privacy through Trusted Execution Environments & Regulation Compliance
As the processing of algorithms is done in a decentralized environment in which large numbers of infrastructure providers lend their computational power, some privacy concerns arise. The AI innovators must be comfortable with the fact that their algorithms will be running on different peers around the network, and the data providers should be the only ones who truly have a right over their data. This means that the owners of the algorithms and datasets must have the ability to make their intellectual property private. In order to achieve this goal, the data and algorithms must be kept encrypted at all times. This part of the process is straightforward; a more delicate part is running algorithms over the data in a manner which does not leak the source code or the data. On the other hand, however, infrastructure providers should rest assured that the algorithms they execute will not exploit and compromise their machines.
Fortunately, the technology that empowers such security features is already available, and is referred to as the Trusted Execution Environment. A trusted execution environment is also called an enclave, and is a privileged part of a processor’s microarchitecture, to which not even the operating system has access. Therefore, an enclave can safely keep and process information that is regarded as secret, without interference from the outside. It also has a limited set of capabilities, so its capacity to exploit programs from the outside is restricted.
To execute an algorithm while at the same time preserving privacy, a service provider must fetch the encrypted data and the encrypted algorithm. Decryption must be done only inside the enclave. To do so, the enclave will have to fetch the decryption keys securely from a service within the platform, called a secret store. The keys will be passed into the enclave, and then execution will begin. Once results are available, they will next be passed to their owner, following the phase in which the appropriate rewards are distributed.
In light of the increasing emphasis on giving users control over personally-identifiable information, Openfabric strives to adhere to the GDPR regulations, even though their application in decentralized environments has not yet been standardized. This means that the beneficiaries of the platform will have full control over what data they disclose. A successful way for implementing such a feature is to encrypt the data that a user chooses to share and then keep the encryption key to themselves, as well as in the platform, where it is encrypted once again. When the platform encrypts the key to the personal data, it distributes the decryption key amongst its users, so that no single peer can obtain it. If a user decides to stop sharing parts of their data, then all they have to do is simply prompt the network to delete the key which grants access to the key that was used to encrypt the personally-identifiable information.
Enterprise Integration
As mentioned before, one of Openfabric’s main goals is to democratize access to AI solutions, and so the platform must be able to include everyone, from the lone individual to the recently-founded startup, to the large enterprise. We believe that companies should also be able to experience the advantages of the newest technologies. Usually, they are not the early adopters of cutting edge technology, mainly because the process is hindered by bureaucratic hurdles. Nevertheless, Openfabric is designed to offer enterprise integration by providing a set of layers through which enterprises can interact with the ecosystem. The first layer is the cognitive layer, which is used to form a connection between the enterprise and the ecosystem through providing a set of microservices, connectors and REST endpoints. The second layer, known as the service layer, is used for data ingestion and normalization, as well as setting up workflows. This layer is highly useful for automating business processes that contain steps of complex AI computation.
Outro
The Openfabric team is extremely excited to have presented you with a summary of the novelties in our platform. Because summaries tend to naturally be a technique of lossy compression of information, we also strongly encourage you to visit us and read our technical whitepaper. And while you’re there, be sure to stay up-to-date with our progress by subscribing to our newsletter, and keep in touch with us so that we can fulfill the promise of the AI revolution together!
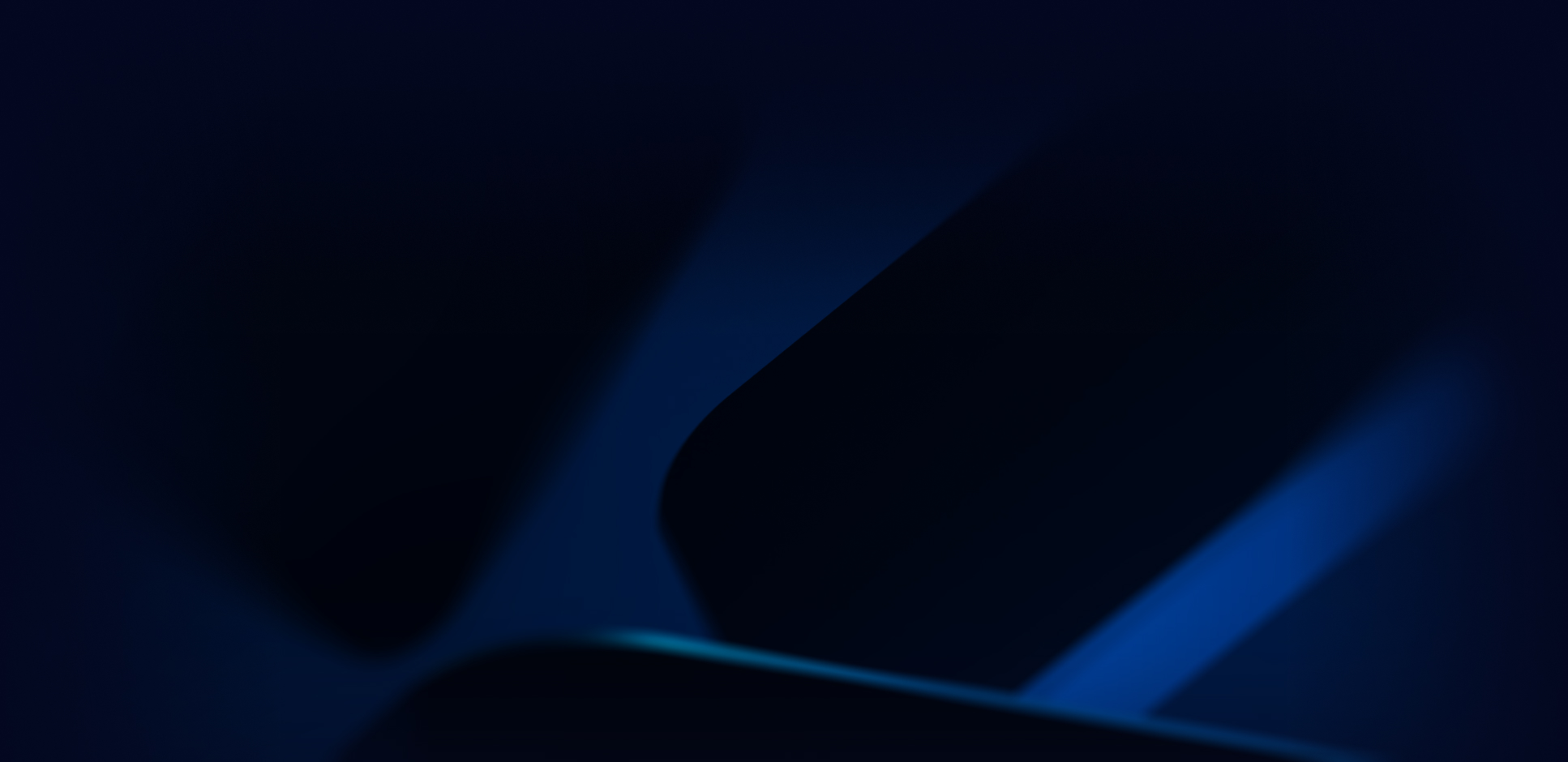