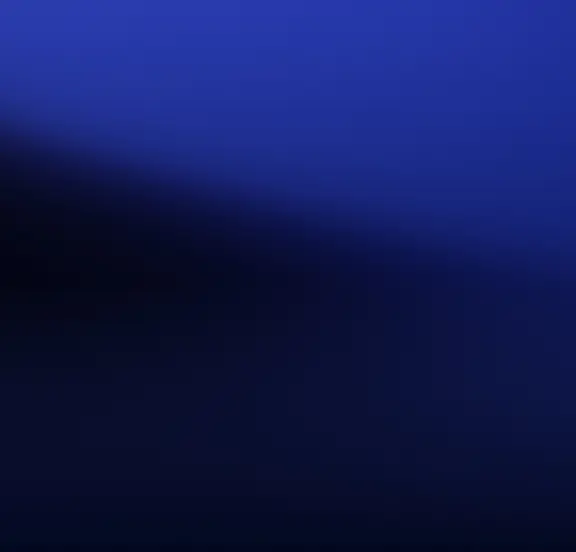
November 29, 2024 7 minutes read
AI Agents and their Transformative Applications in 2025
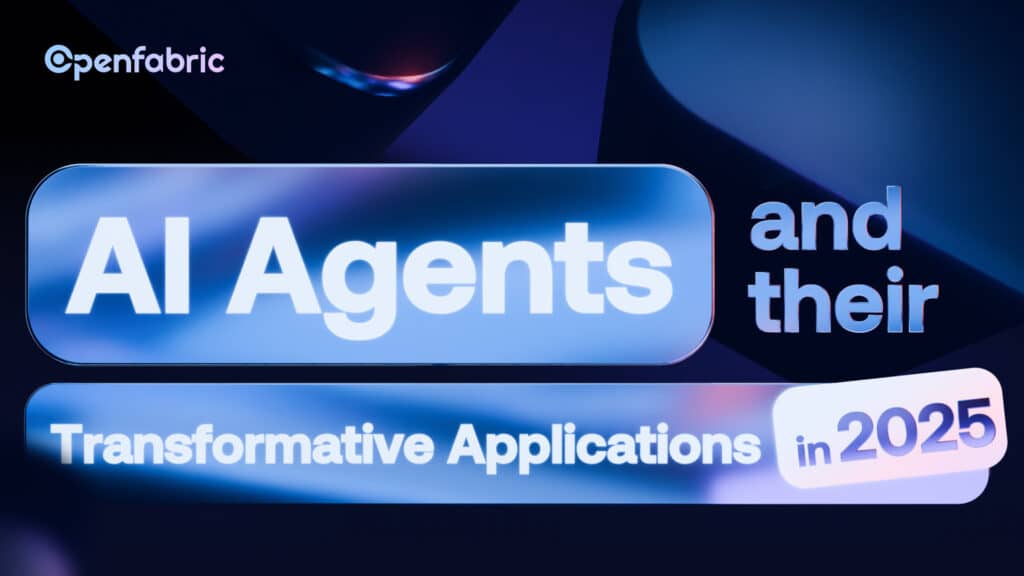
The wide adoption of generative AI in 2022 made people more familiar with the concept of AI. Generative AI also paved the way for AI agents. While generative AI like ChatGPT requires human input, AI agents work almost independently with minimal or no human assistance. As 2024 rounds up, it is clear that in 2025, we will see more AI agents and their transformative applications to ease an average human life.
But what are these AI agents?
Artificial Intelligence (AI) agents refers to a program or system that is completely capable of performing tasks independently and autonomously without the help. In addition, this system also has the ability to learn from its experiences which allows it to improve its performance over time.
These AI agents are used in various industries for problem-solving, decision making and other complex tasks, without the aid of an individual. Independently, they solve problems and learn from it to optimize themselves for further tasks.
AI agents are complicated systems. In order to fully maximise the potentials therein, there are certain things that should be known. In this article, we will be looking at the intricacies surrounding AI agents and see their transformative applications in 2025.
Let’s get started
Types of AI agents
There are five types of AI agents. These agents have the basic ability of functioning autonomously. However what makes them different is the level of their intelligence, and how they work. Based on these two factors, there are five types of AI models.
Simple reflex agent
This type of AI agent works based on the currently available information and not on history or past information, no matter how relevant. The function of this agent is based on the condition-action rule. With this rule, there is a condition or state that is linked to the action such that the action is taken only when the condition is right, otherwise the action is not taken at all.
In a fully observable environment, these agents are successful. On the other hand, they are unsuccessful if the environment is not fully observable. Therefore, their intelligence is very limited. Examples include Home dirty cleaner, thermostat and so on.
Model-based reflex agent
The model-based agent, just like the simple reflex agent, works based on a condition-action rule. However, it differs in that the model-based agent can work in a partially observable environment. It does this by tracking the situation and using a particular model related to the knowledge of the world to operate.
There are two important factors of this model. These are:
- Model: It provides the knowledge and understanding of how different things in the surroundings happen. By doing so, the current situation can be studied and a condition can be created. The agency performs its actions based on this model.
- Internal State: The Internal State represents the current state based on perceptual history. The agent monitors the internal state, and keeps adjusting it based on the percept history.
Example is a vacuum cleaner that cleans diets but knows how to navigate obstacles in its path.
Goal-based agents
These AI agents are goal oriented and as such, a simple knowledge of their environment is not often enough. The agent needs to know its goals.
This agent functions by analysing the current situation and choosing the best possible action in order to achieve that set goal. The idea of choosing the best possible action is to reduce the time for that goal to be achieved.
The carries out considerations, called searching and planning, to identify and map out each possible scenario. This extra ability makes them more proactive than the previous agents. Example is a game playing AI whose goal is to win the game.
Utility-based agent
Utility-based agents are similar to model-based agents in that they are also goal based. However with utility-based agents, there is an extra ability to choose the best possible action to take when there are multiple alternatives. In other words, when there are multiple conditions presented, utility-based agents are the best because they have the ability to run the scenarios or each condition and identify which one is best to efficiently achieve their goals.
A good example is a delivery drone that optimizes factors like delivery time, energy consumption, and customer satisfaction to make delivering packages to customers more efficient.
Learning agent
The learning agent is an AI agent that has the capability to learn from past experiences and takes actions or decisions based on the knowledge acquired. It gains basic knowledge from the past and uses that learning to automatically act and adapt.
There are four components in the learning agent and they include:
- Learning element: It is the part that makes improvements by learning from the environment.
- Critic: Critic provides feedback to the learning agent giving the performance measure of the agent in comparison to the fixed performance standard.
- Performance element: It chooses the external action.
- Problem generator: This is responsible for suggesting actions that will lead to new and informative experiences.
What is the Future of AI Agents
AI agents have continued to be a transformative tool in the modern world. So far, we have seen their applications in our day-to-day activities and even in business. Nowadays, we have home and office assistants, and artificial intelligence powered tools that make our lives easier. Some of these tools are sophisticated enough to do the heavy-lifting while we sit back and focus on other aspects of our lives. Or it could just be to take a much needed break.
In the future, we expect to see more applications of AI agents in the world. In our world today, human-decision making is mainly by intuition and not by proper research and important considerations. This often leads to poor outcomes and in some cases loss. Already, AI agents are evolving to come in where human based decision making fails. AI agents make decisions that are backed up by facts, data and history dated as far back as possible. This will reduce the risk of inaccuracy in finance, medicine, security and other relevant industries.
AI agents will also see tremendous use in learning. The system of learning we all have adapted to is a one-size-fits-all system. Here, learning plans and models are not tailored specifically as would better suit individual intellectual strengths. AI agents on the other hand can create learning models that are tailored specifically for individuals. Time and research has shown that this method of learning is more suitable and carries everyone along.
With time, we expect a world where AI agents understands the relationship between cause and effect therefore creating opportunities for everyone and opening the door for more use cases in various industries
Limitations of AI Agents
Despite their transformative power, AI agents still have some limitations that prevent them from being utilized the way they should be. Knowing these limitations only help us to identify where we can help improve AI agents and what we should expect from them.
- Data privacy concerns: due to the high amount of data they are exposed to, there are concerns about how this data is used, and what it should be used for. Furthermore, some companies may exploit this data for personal gains without an agreement from the owners
- Security risks: AI agents are targets of cyber security attacks due to the volume of information they store and learn from.
- Performance and complexity: some AI agents are not evolved enough to handle certain complex tasks. This often results in poor performance and lack of satisfaction.
- Poor adoption: statistics show that AI agents have been poorly adopted over the years. This is a huge limitation as AI agents need data from consumers to evolve and provide a better service to its users. Without the data, AI agents will not grow.
Conclusion
AI agents offer numerous benefits such as efficiency and autonomous but precise decision making. They help minimize losses and mistakes due to their availability to historical data and patterns. The most important feature of AI agents is their capability to learn from previous experiences and optimize their performance accordingly. A wide adoption of AI agents should be encouraged to allow this technology to serve us better.
Stay ahead of the curve with more updates on our WEBSITE.
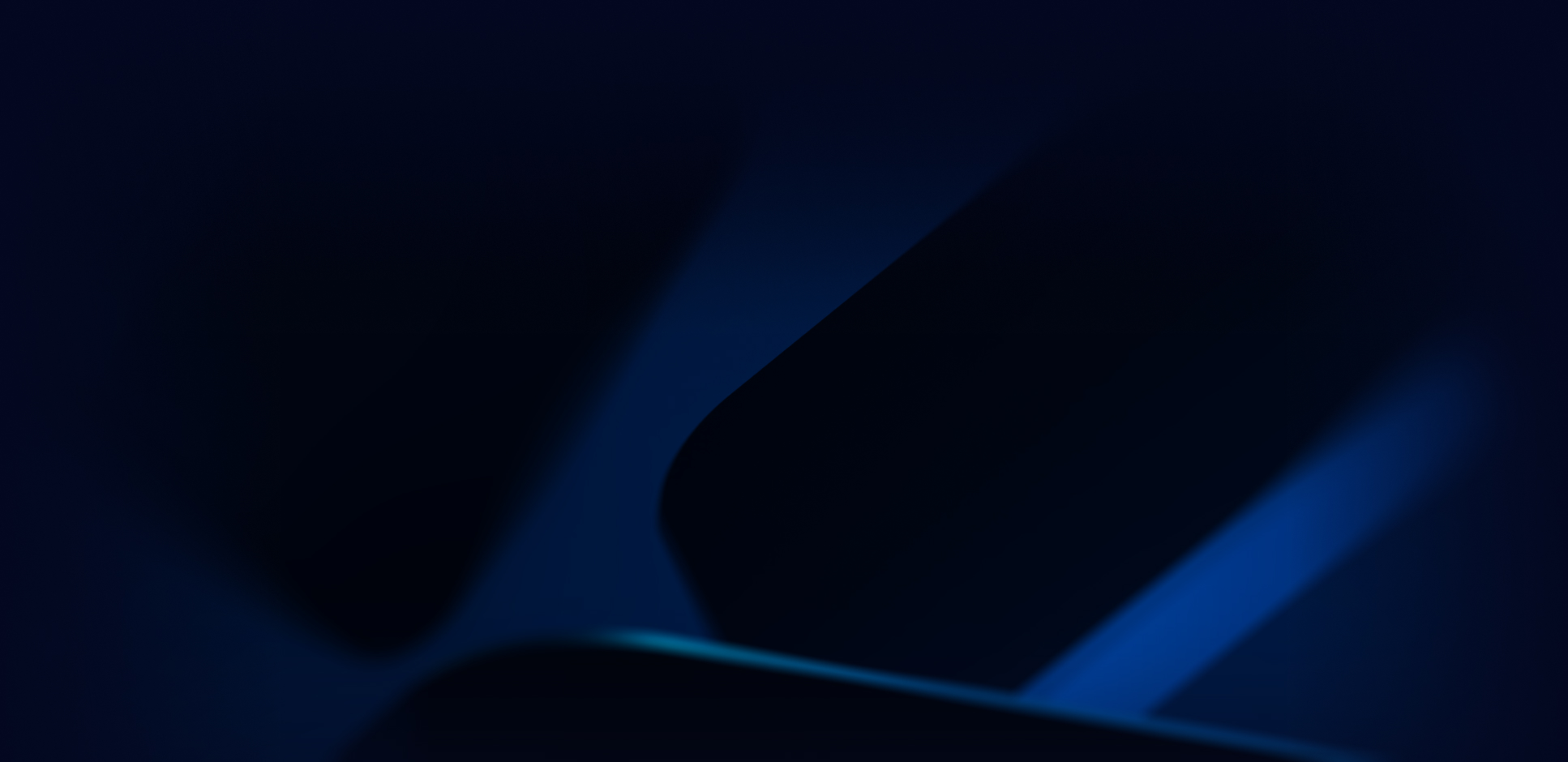