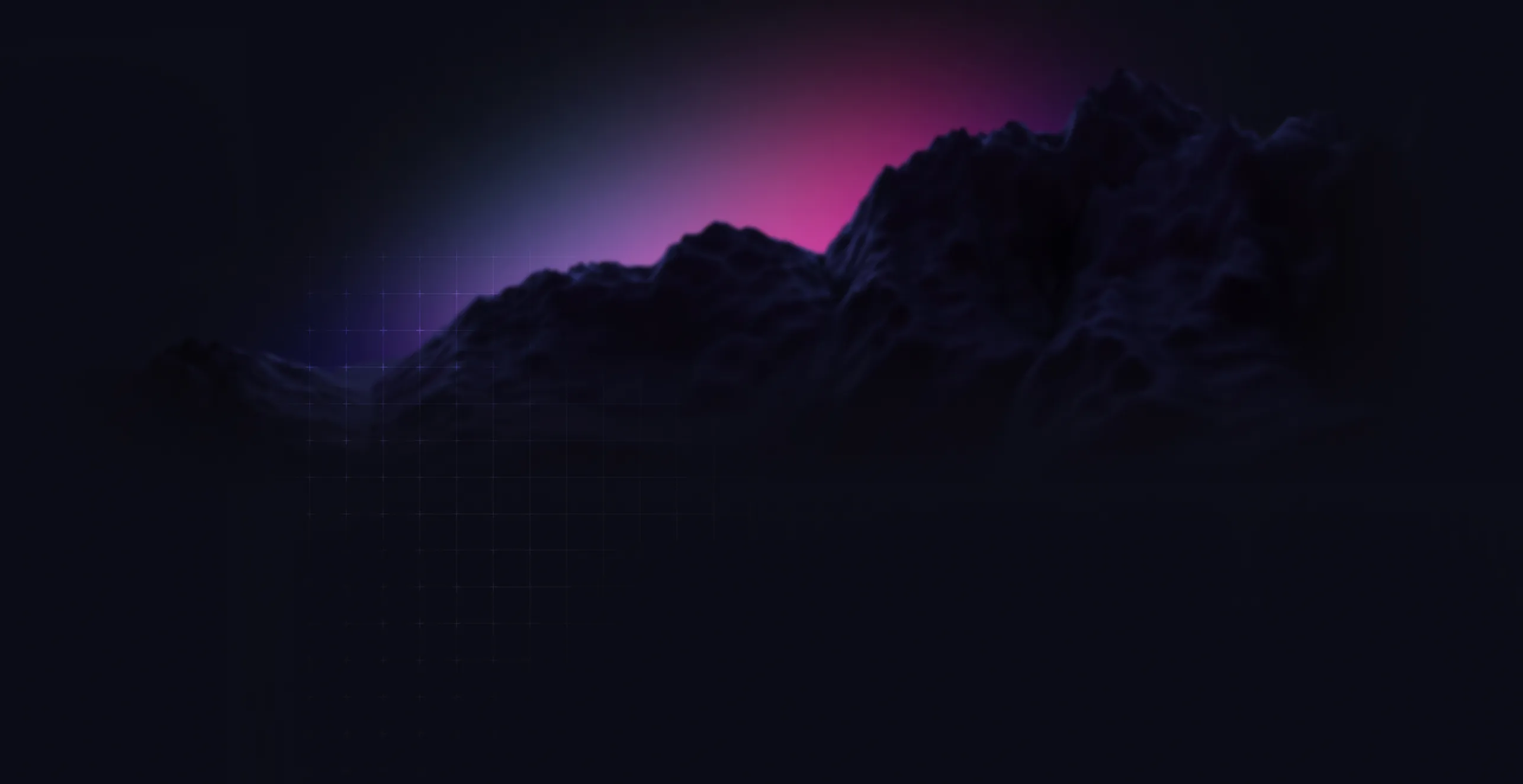
What’s Next In Artificial Intelligence?
April 12, 202412 minutes read
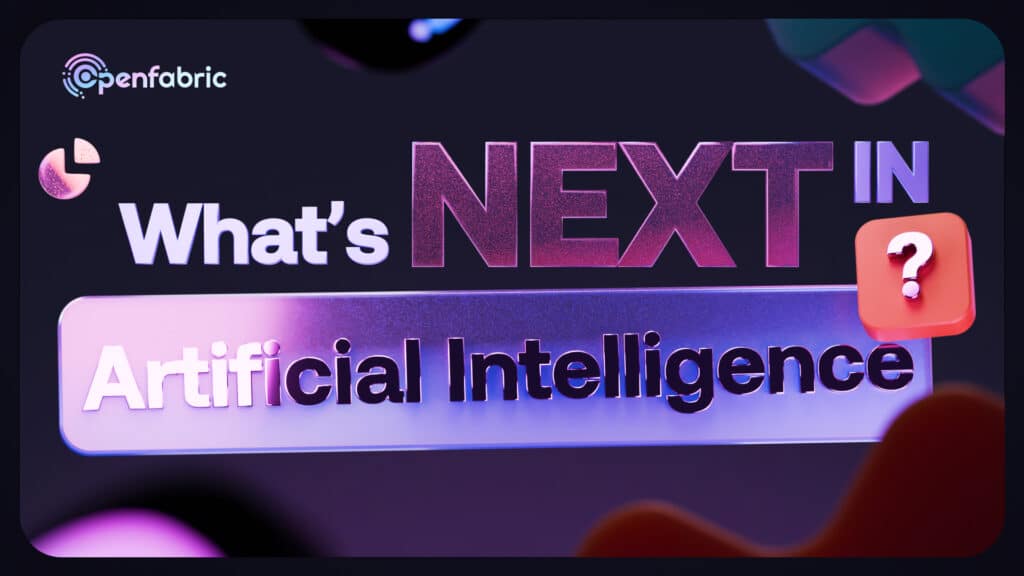
Openfabric AI. Yes, without mincing words that is an outright answer. A plethora of reasons back this claim and later in this article AI enthusiasts will understand how AI and Openfabric are synonymous. Over the last few years, Artificial Intelligence has evolved quite quickly. It is gaining grounds in core parts of our daily lives. The current buzz around Artificial Intelligence finds us asking important questions and one of them happens to be What’s next in AI? Let’s get into this topic and see reasons why Openfabric happens to be the best answer. We will address the following and the role Openfabric is actively playing to make sure Artificial Intelligence is at the forefront of technological advancements. These 5 points will be discussed:
- Continued Advancements in Deep Learning
- Ethical Artificial Intelligence and Responsible Innovation
- Explainable AI and Trustworthiness
- AI at the Edge and Decentralized Computing
- Lifelong Learning and Adaptive AI Systems
Continued Advancements in Deep Learning
Deep learning remains the propelling force behind many recent AI breakthroughs. Researchers are continually enhancing deep learning models to expand their capabilities. Transitioning from traditional neural networks, these efforts include attention mechanisms, transformers, and self-supervised learning. These advancements hold promise for various fields such as natural language processing, computer vision, and robotics. Talking of deep learning, ongoing research efforts aim to enhance its capabilities. Attention mechanisms, for example, enable models to focus on relevant information while filtering out noise. This enhances their ability to understand complex data. Additionally, transformers have emerged as a powerful architecture, facilitating efficient processing of sequential data. Such advancements pave the way for more accurate and versatile AI systems.
Moreover, self-supervised learning techniques enable models to learn from unlabeled data. This reduces the need for extensive labeled datasets, making AI development more accessible and cost-effective. As a result, researchers can train models on vast amounts of readily available data, improving their performance across various tasks. These advancements in deep learning have significant implications for real-world applications. In natural language processing, advanced models can generate more coherent and contextually relevant responses. This enhances the user experience in chatbots, virtual assistants, and language translation services. Talking of what’s next in Artificial Intelligence, in computer vision, deep learning enables more accurate object detection and image recognition. This is crucial for the growth of applications like autonomous vehicles, surveillance systems, and medical imaging.
Complex Technologies and Artificial Intelligence
Talking about complex technologies and what’s next in artificial intelligence for them, in the field of robotics, deep learning facilitates more sophisticated decision-making and control algorithms. Robots can navigate complex environments, manipulate objects with greater precision, and interact more effectively with humans. This opens up opportunities for automation in industries such as manufacturing, healthcare, and logistics. As these technologies continue to evolve, the potential for AI applications will only grow. Deep learning algorithms will become more efficient and adaptable, enabling AI systems to tackle increasingly complex tasks. From understanding human speech to interpreting visual data and controlling physical machines, the possibilities are endless.
The future of Artificial Intelligence lies in the continued advancements in deep learning. By improving models and expanding their applications, researchers are paving the way for more intelligent and capable AI systems. Whether it’s enhancing communication, solving complex problems, or augmenting human abilities, deep learning will play a key role in shaping the next phase of AI innovation.
Ethical Artificial Intelligence and Responsible Innovation
The growth of artificial intelligence (AI) has thrown us into an era where the ethical dimensions of technology wield significant influence over our daily lives. Gone are the days when Artificial Intelligence was solely a matter of technological advancement; now, it’s a moral imperative to ensure that AI is developed and utilized responsibly. It’s only normal or expected that Ethical AI is a part of what’s next in artificial intelligence.
One of the most pressing concerns surrounding AI is its potential for bias. AI systems are often trained on datasets that reflect societal biases, leading to discriminatory outcomes, particularly in areas such as hiring, lending, and law enforcement. These biases can perpetuate existing inequalities and marginalize already vulnerable populations. Addressing bias in AI requires proactive measures to identify, mitigate, and prevent discriminatory algorithms. Privacy is another ethical minefield in the realm of AI. As AI systems collect and analyze vast amounts of personal data, concerns about data privacy and security have escalated. Individuals are rightfully worried about how their data is being used and whether it’s being handled in accordance with ethical principles. Striking a balance between the utility of AI-driven insights and the protection of individuals’ privacy rights is paramount to building trust in AI technologies.
Mitigation of Negative Effects
Moreover, the societal impact of AI cannot be understated. From job displacement to shifts in power dynamics, the widespread adoption of AI has far-reaching consequences. It’s imperative to consider the broader societal implications of AI deployment and to actively mitigate any negative effects. This requires engaging with diverse stakeholders, including policymakers, ethicists, and affected communities, to ensure that AI serves the collective good. In response to these ethical challenges, there’s a growing recognition of the need for ethical AI frameworks and guidelines. These frameworks provide a roadmap for developers and users to navigate the ethical complexities of AI, guiding them in making decisions that prioritize fairness, transparency, and accountability. By adhering to ethical principles, we can harness the power of AI to drive positive change while minimizing harm.
Fortunately, there are initiatives underway to promote ethical AI development and deployment. Organizations, both public and private, are investing in research, education, and policy advocacy to advance the cause of ethical AI. These efforts are essential for fostering a culture of responsibility within the AI community and ensuring that AI technologies are developed and used in ways that align with our values and aspirations. As we look to the future, it’s clear that ethical considerations will continue to shape the trajectory of AI innovation. By proactively addressing ethical challenges and prioritizing the well-being of individuals and society, we can unlock the full potential of AI to improve lives and drive progress. Ethical AI isn’t just a buzzword; it’s a moral imperative that demands our attention and action. You can read more about Ethical Consideration in Artificial Intelligence on Openfabric’s blog.
Explainable Artificial Intelligence and Trustworthiness
A critical aspect that has garnered significant attention in Artificial Intelligence is the imperative for AI systems to clarify their decisions and actions. This necessity becomes even more pronounced in domains where the consequences of AI-generated outputs hold substantial weight, such as in healthcare and finance. The absence of clear and coherent explanations for AI-driven outcomes can breed skepticism, erode trust, and impede the adoption of these technologies. To confront this challenge, researchers and practitioners are actively engaged in devising methodologies to render AI models more interpretable and transparent. One approach gaining traction is the integration of attention mechanisms, which enable AI systems to highlight pertinent features or components of data that influence their decisions. By emphasizing relevant information, attention mechanisms not only enhance the understanding of AI processes but also facilitate users’ comprehension of how decisions are reached.
Another technique that contributes to the interpretability of AI models is feature visualization. Through this method, users are provided with visual representations that elucidate the factors driving AI-generated outcomes. By offering insights into the underlying features that contribute to decisions, feature visualization empowers users to discern the rationale behind AI-driven actions, thereby fostering trust and confidence in these systems.
On clarity and simplification…
Model distillation has emerged as a valuable strategy to enhance the transparency of AI models. This process involves simplifying complex AI architectures into more comprehensible versions, while preserving their accuracy and performance. By distilling intricate models into more accessible forms, developers can offer users a clearer understanding of AI operations, thereby mitigating concerns regarding opacity and unpredictability therefore giving us a clearer picture on what’s next in AI.
The integration of explainable AI not only serves to engender trust among users but also plays a pivotal role in navigating regulatory frameworks and compliance requirements. Clear and transparent explanations of AI-generated outputs facilitate regulatory oversight and ensure adherence to ethical guidelines and standards. Moreover, by promoting transparency and accountability, explainable AI fosters collaborative partnerships between AI developers, end-users, and regulatory bodies, thereby driving responsible and ethical deployment of AI technologies.
Summarily, the advancement of explainable AI techniques holds profound implications for the future trajectory of AI development and deployment. By leveraging approaches such as attention mechanisms, feature visualization, and model distillation, stakeholders can enhance the interpretability and transparency of AI systems, particularly in critical domains like healthcare and finance. Through the provision of clear and coherent explanations, explainable AI not only increases trust and confidence among users but also facilitates regulatory compliance and ethical deployment, paving the way for the responsible integration of AI technologies into diverse applications and sectors.
AI at the Edge and Decentralized Computing
The future of AI is gearing up for a big change as it heads towards something called “edge computing.” It’s like moving the brain closer to where the thinking needs to happen. This change is going to make a huge difference in how we use AI. Instead of relying on faraway servers to do all the heavy lifting, edge computing lets us do the thinking right where the data is created. Think about it this way – devices like your smartwatch or your smartphone can do smart stuff without having to ask for help from servers miles away.
But this shift isn’t all smooth sailing. There are hurdles we need to jump over, like making sure AI models can run on devices that don’t have a lot of power or memory. It’s like teaching a big brain to fit into a small head. But if we can figure out how to do this, it opens up a whole new world of possibilities. With AI at the edge, things can happen really fast. We’re talking about making decisions in real-time, like making sure a self-driving car knows when to stop or go, or keeping track of machines in a factory to make sure they’re working smoothly.
And then there’s what is called “decentralized AI,” which is like spreading out the smarts across a bunch of devices. This is a big deal because it means we can keep things private, reduce delays, and handle more stuff without a hitch. With decentralized AI, the data gets processed right where it’s created, which means we don’t have to worry about sending sensitive information over the internet. It’s like having a secret meeting in your own backyard instead of shouting your secrets across town.
Decentralization and faster computing
And you know those times when you’re waiting for something to load on your computer, and it feels like forever? Well, decentralized AI helps with that too. By spreading out the work, we can get things done much faster. It’s like having a bunch of friends help you with your homework instead of trying to do it all by yourself. Plus, as more devices join the network, we can handle even more work without slowing down.
So, to sum it all up, the future of AI is all about bringing the smarts closer to where they’re needed and spreading them out across a bunch of devices. Sure, there are challenges along the way, like making sure AI can work on smaller devices, but the possibilities are endless. With AI at the edge and decentralized architectures, we’re looking at a future where things happen faster, are more private, and can handle whatever we throw at them. And that’s pretty exciting.
Lifelong Learning and Adaptive AI Systems
Current AI systems face limitations in adaptability and continuous learning. They struggle to learn from new data and experiences, hindering their ability to change over time. However, ongoing research in lifelong learning, meta-learning, and continual learning aims to overcome these challenges. These efforts focus on creating AI systems capable of acquiring and refining knowledge throughout their operational lifespan.
One area of focus in advancing AI is lifelong learning. This approach enables AI systems to accumulate knowledge and skills gradually, much like humans do. Instead of being trained on static datasets, these systems continuously learn from new information they encounter. This capability is crucial for applications requiring adaptability and flexibility, such as personalized education. Another promising avenue is meta-learning, which involves developing AI models that can learn how to learn. By understanding the learning process itself, these systems become more efficient at acquiring and applying knowledge. Meta-learning empowers AI to adapt quickly to new tasks and environments, making it invaluable for domains like autonomous vehicles.
Continual learning is also gaining traction as a means to enhance AI adaptability. Unlike traditional machine learning approaches, continual learning allows AI systems to learn incrementally over time. They can update their understanding as new data becomes available, ensuring relevance and accuracy in dynamic environments. This capability holds significant potential in personalized healthcare, where AI must stay current with evolving medical knowledge and patient data.
Moving from traditional AI to adaptive AI systems
Adaptive AI systems hold promise across various domains. In personalized education, these systems can tailor learning experiences to individual students’ needs and preferences. By continuously analyzing performance and adjusting instructional content, they foster more effective and engaging learning environments.
In autonomous vehicles, adaptive AI is critical for navigating complex and unpredictable road conditions. These systems must continually learn from real-world driving experiences to improve safety and efficiency. By adapting to changing environments and evolving traffic patterns, they enhance overall driving performance and reliability. In personalized healthcare, adaptive AI can revolutionize patient care by personalizing treatment plans and interventions. These systems analyze vast amounts of medical data to generate tailored recommendations based on individual health profiles. By adapting to each patient’s unique circumstances and responding to new research findings, they optimize outcomes and minimize risks.
systems represents a significant step forward in the field. It allows AI to evolve alongside changing circumstances and emerging challenges, unlocking new possibilities for innovation and progress. By embracing lifelong learning, meta-learning, and continual learning, we pave the way for AI systems that are not only intelligent but also adaptable and resilient in a constantly changing world.
Openfabric And AI
Openfabric is the next frontier in AI, positioning itself as an innovative AI protocol for building and connecting AI Apps.. With its seamless interface and diverse range of applications, Openfabric stands out as a game-changer in the AI landscape. Transitioning from traditional models, it offers a user-centric platform where individuals can access, customize, and implement AI solutions tailored to their needs.
Users experience a streamlined process, from browsing to installation, fostering a user-friendly environment. Openfabric’s curated selection ensures quality and relevance, empowering users to find solutions that precisely match their requirements. As users engage with the platform, they encounter a dynamic ecosystem, continuously enriched with new applications and updates. Moreover, Openfabric facilitates collaboration among developers, fostering a vibrant community where innovation thrives. Developers find a supportive environment to showcase their creations and receive feedback, driving iterative improvements. This collaborative spirit propels the platform forward, ensuring a constant influx of fresh ideas and solutions.
Openfabric is putting together supercomputers that are spread out, aiming to give more people access to AI. This means AI development will be cheaper, safer, and clearer. It also means AI startups will save lots of money on computers.
Furthermore, Openfabric emphasizes accessibility, offering a range of pricing options to accommodate diverse budgets. This inclusivity democratizes AI, making advanced technologies accessible to individuals and businesses of all sizes. In essence, Openfabric’s rise marks a paradigm shift in AI accessibility and utilization. Through its intuitive platform, collaborative community, and affordability, it paves the way for widespread AI adoption, heralding a new era of innovation and empowerment.
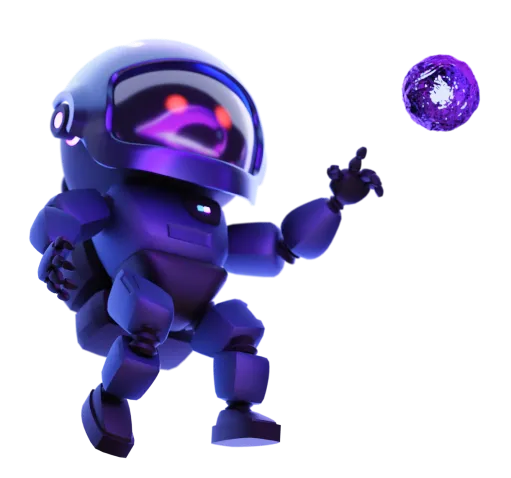
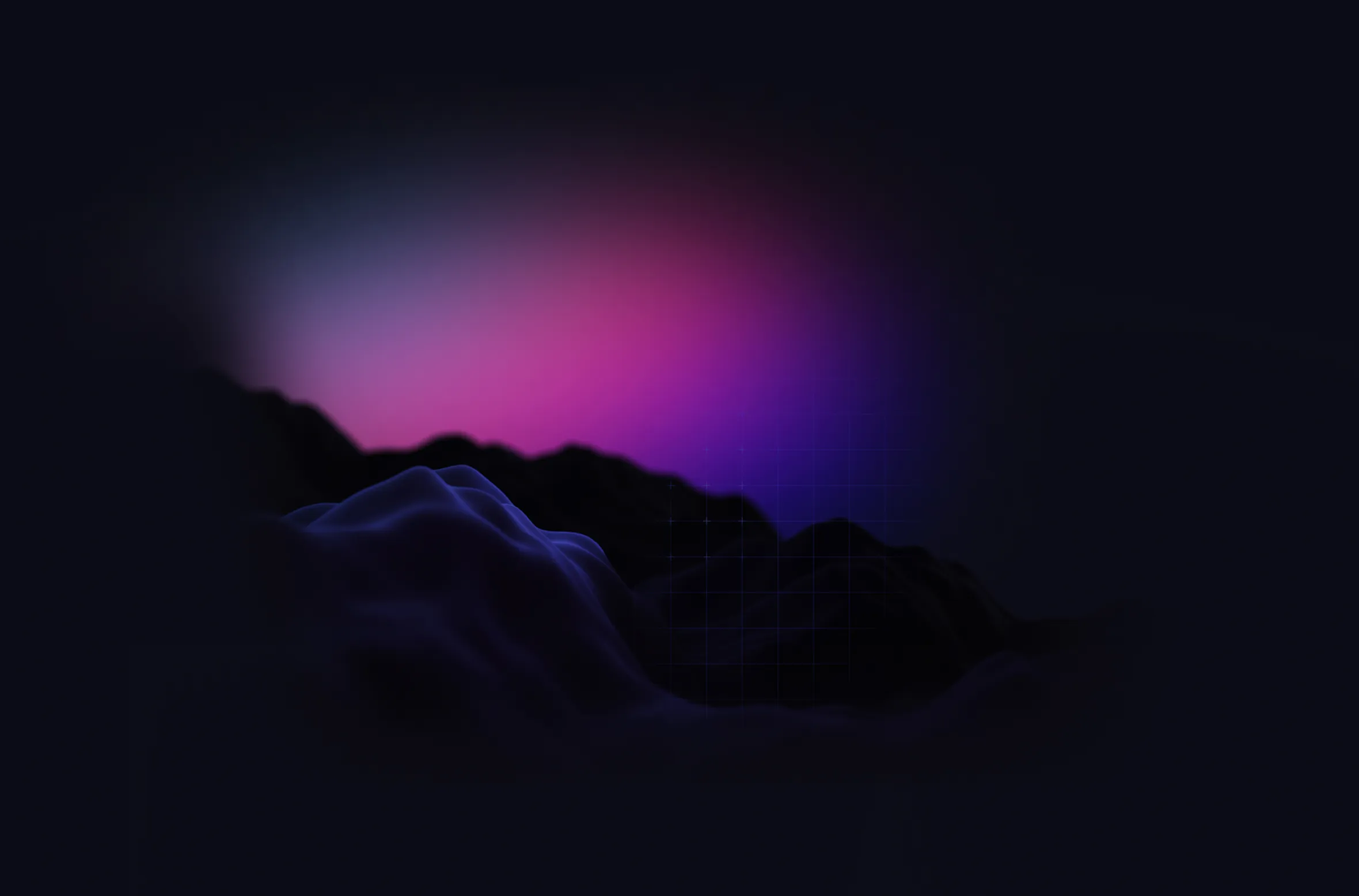
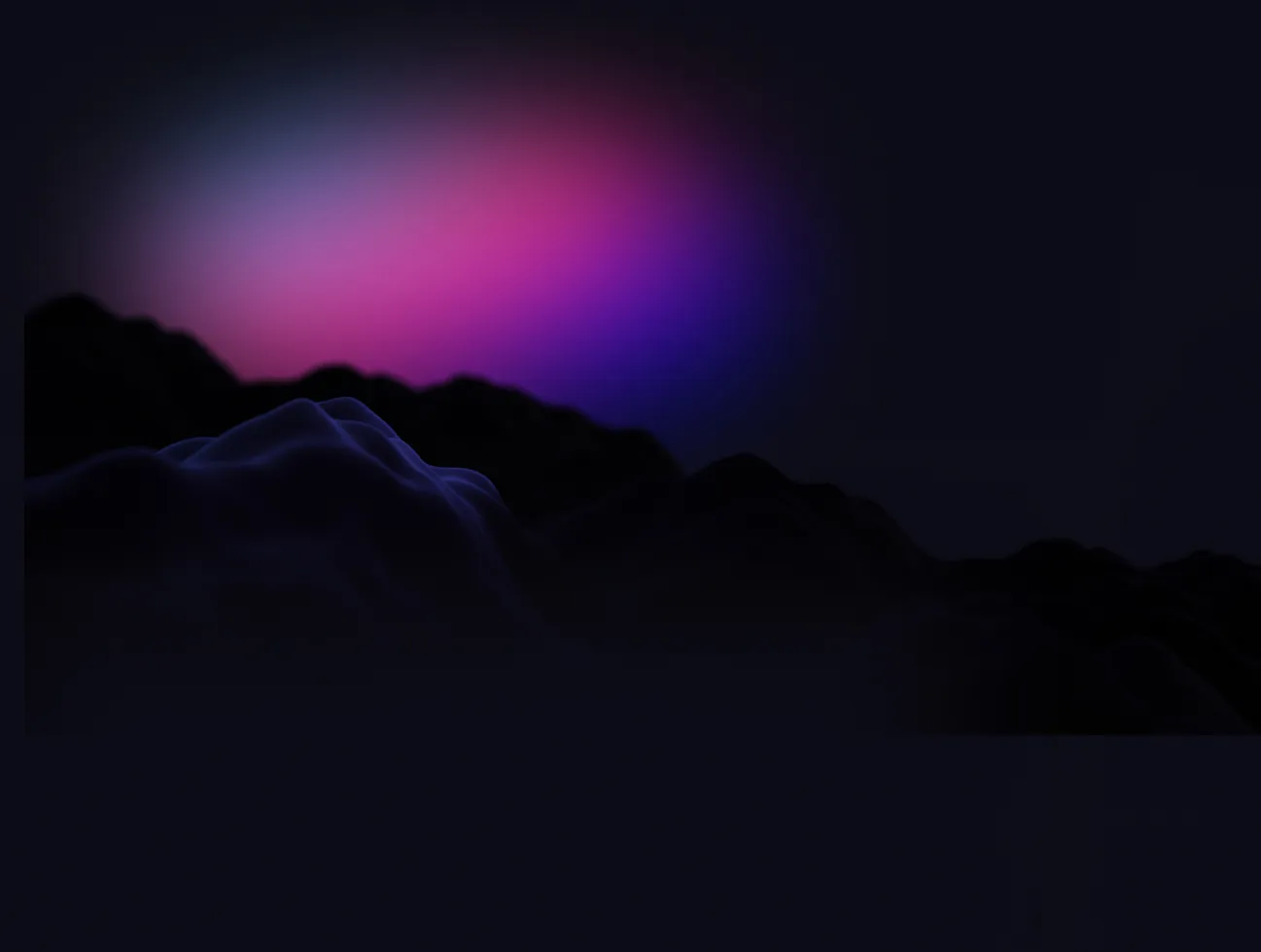